Computer vision in aircraft quality control and damage detection

December 6, 2024
Explore how computer vision and models like Ultralytics YOLO11 can enhance aircraft quality control and damage detection.
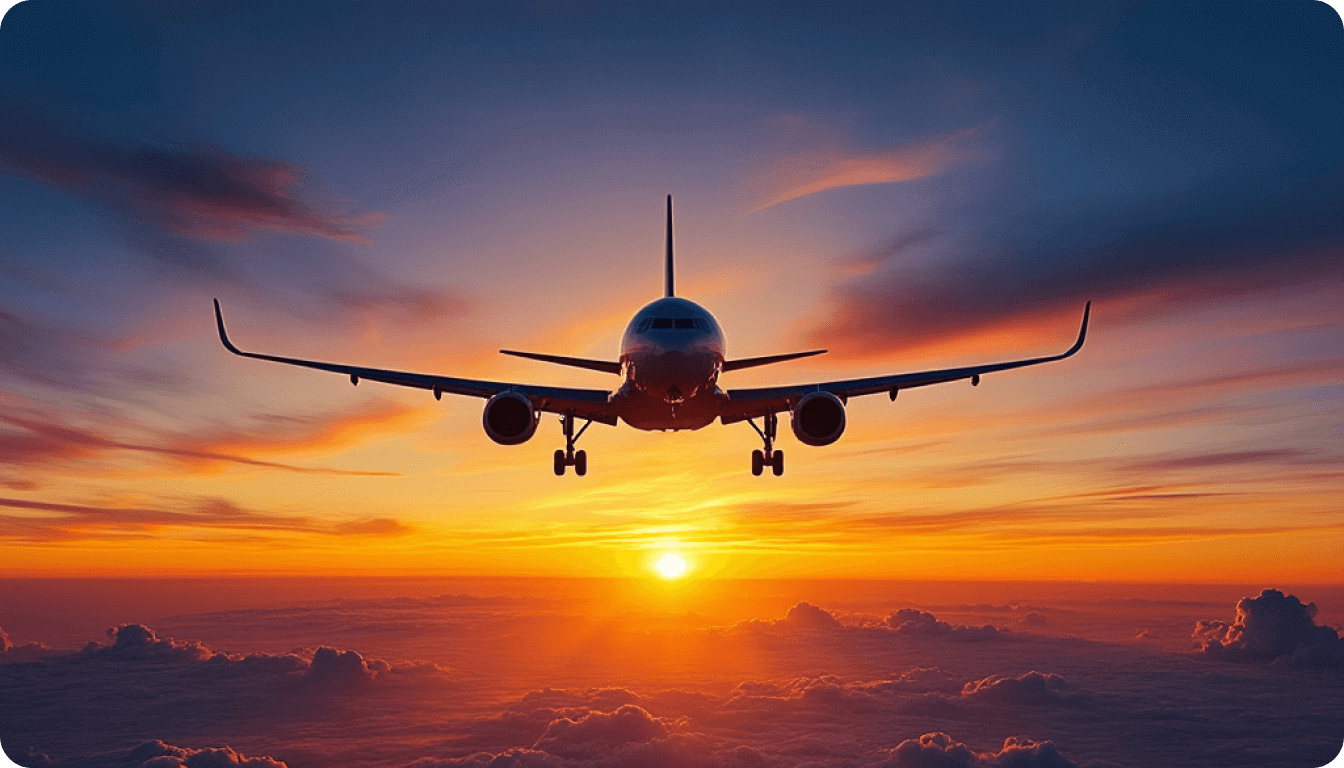
December 6, 2024
Explore how computer vision and models like Ultralytics YOLO11 can enhance aircraft quality control and damage detection.
Aircraft maintenance is the backbone of aviation safety, ensuring planes remain operational and compliant with stringent regulatory standards. However, traditional inspection methods, like manual checks for dents or corrosion, can be time-consuming and prone to human error. As the aviation sector expands, the need for innovative solutions becomes more critical.
Recent advancements in aviation technology demonstrate the transformative potential of AI and computer vision. Tools designed to streamline engine inspections have reportedly reduced inspection times by up to 90%, showcasing how these innovations are reshaping aircraft maintenance processes. Such developments are enhancing quality control, minimizing downtime, and setting new benchmarks for safety standards in the industry.
Let’s explore how vision AI and computer vision models like Ultralytics YOLO11 can support aircraft quality control and its applications during different steps of aircraft quality control.
Computer vision, a branch of AI, enables machines to analyze and interpret visual data with remarkable accuracy and efficiency.
In the aviation industry, this technology can become an ally in shaping how aircraft are inspected, maintained, and repaired. By processing high-resolution images and videos captured from drones, borescopes, or fixed cameras, computer vision models can identify structural defects, corrosion, or other forms of damage on an aircraft's surface and components, resulting in a great step forward toward improving operational efficiency and ensuring compliance with strict safety standards.
The integration of computer vision models like YOLO11, with advanced capabilities such as object detection, instance segmentation, and oriented bounding box (OBB) detection, allows for real-time analysis of complex aircraft surfaces. These tools can detect dents, cracks, and other anomalies that are often difficult to identify with the naked eye, especially in areas with limited accessibility like engine components or undercarriages.
To this effect, computer vision plays an exciting role when it comes to real-time damage detection during inspections.
Traditional methods often rely on manual, time-intensive visual checks, which can lead to inconsistencies and missed issues. Computer vision, in contrast, provides a consistent and scalable solution by automating these processes, allowing operators to focus on areas of concern flagged by the system while optimizing the inspection process and reducing the risk of oversight.
So let’s take a look at how computer vision can help with aircraft maintenance.
Aircraft maintenance is a multifaceted process, and vision AI solutions are at the forefront of these innovations, offering diverse applications tailored to aviation needs.
One of the most impactful applications of computer vision in aircraft inspections is real-time defect detection. Traditional manual inspections can be labor-intensive and rely heavily on human expertise, which can introduce variability and errors.
Computer vision models can build on this process by analyzing high-resolution images or video streams to detect anomalies such as dents, scratches, and corrosion. Advanced algorithms, including segmentation and feature extraction, enable precise identification of these defects even in complex surfaces like engine blades or fuselage panels.
Detecting corrosion and paint deterioration is of high importance when it comes to maintaining aircraft integrity. Computer vision enables early detection by analyzing color variations, surface textures, and patterns indicative of wear. Advanced preprocessing tools can segment areas affected by rust or peeling paint, allowing for targeted maintenance.
Using UAVs (drones) for surface inspections further enhances the capabilities of computer vision systems. These devices capture high-resolution images of hard-to-reach areas, such as wingtips or rudders, enabling comprehensive analysis without requiring complex scaffolding or human intervention.
Structural components, such as fuselages and wings, are subjected to significant stress during operation. Computer vision facilitates structural health monitoring by assessing geometric deformations, detecting surface cracks, and evaluating wear.
For example, systems trained on annotated datasets can differentiate between normal wear patterns and critical issues requiring immediate attention.
Engine blades endure extreme temperatures and rotational stresses, making regular inspections critical. Computer vision can facilitate the detection of defects such as micro-cracks, blade tip wear, and pitting corrosion. Algorithms like U-Net or advanced GAN models refine these detections by enhancing image clarity and eliminating noise.
Moreover, computer vision approaches are highly effective for assessing damage in borescope images, as they provide a high level of accuracy. This ensures that even minor defects, which could escalate into critical failures, are promptly identified.
The use of AI has become more and more used across various industries and that of aircraft management is no exception. And while there are countless technologies and computer vision solutions in the field, YOLO models have been a popular choice.
YOLO11 is the latest of the YOLO series and one of the best object detection models bringing unparalleled computer vision capabilities to the aviation industry.
Supported Tasks Include:
So how can these be applied to the aviation industry? Some key applications include
One of YOLO11’s standout features is its ability to deliver real-time results. The Ultralytics YOLO models can be deployed and integrated into various hardware such as drones or cameras. By scanning an aircraft’s exterior, YOLO11 can detect defects as they occur. This capability allows for rapid response times, minimizing downtime and ensuring continuous operational readiness.
To meet the specific needs of aircraft maintenance, YOLO11 can be trained and tailored to specific needs The models can be trained on high-resolution, aviation-specific annotated datasets featuring real-world scenarios like corroded surfaces, bird strike dents, or structural cracks. Engineers can fine-tune YOLO11 by using these datasets, setting key parameters, and defining defect categories to ensure precise anomaly detection.
The model’s optimized architecture and training pipeline delivers high accuracy while requiring fewer computational resources, enabling fast and efficient learning. By training YOLO11 in this focused manner, aviation engineers can leverage its capabilities to streamline inspections, identify critical damage early, and enhance aircraft safety and operational efficiency.
Integrating computer vision into aircraft maintenance delivers significant advantages, tailored specifically to the unique challenges of using AI in the aviation industry.
While computer vision presents transformative opportunities, its implementation in aviation is not without challenges.
The future of aircraft maintenance is increasingly intertwined with advancements in AI and computer vision. As these technologies evolve, here’s what the aviation industry can anticipate:
AI might have the ability to integrate historical data with real-time inputs from computer vision systems to help predict potential failures. This proactive approach has the potential to reduce unplanned downtime and extend component lifespans.
Future computer vision models may include 3D imaging, enabling more detailed inspections of complex structures. Paired with digital renders of the aircraft, these models could provide real-time updates on an aircraft’s condition, supporting predictive analytics.
Drones equipped with computer vision will become indispensable for inspecting hard-to-reach areas. These UAVs will combine real-time analysis with AI to deliver comprehensive assessments in minutes.
Optimized inspection processes and faster turnarounds will support the industry’s sustainability goals by reducing fuel consumption during maintenance operations.
Computer vision is revolutionizing aircraft maintenance, offering tools that enhance safety, reduce costs, and streamline operations. Models like YOLO11 are setting new benchmarks, delivering unparalleled accuracy and efficiency in damage detection and quality control. As aviation continues to embrace AI-driven solutions, the future holds promise for safer, greener, and more efficient skies.
Discover how YOLO11 is leading the charge in transforming industries like manufacturing. Explore our GitHub repository to learn more about cutting-edge vision AI solutions for aviation and beyond. ✈️