Specialvideo was trying to build a reliable, high-speed quality control process for pizza manufacturers because human inspections couldn’t keep up with producing one pizza every 600ms.
By integrating Ultralytics YOLO models, Specialvideo’s AI food inspection system boosted detection accuracy to over 95% and cut inspection time to under 250ms per pizza.
Pizza manufacturers often need to produce visually appealing, high-quality products at high speed, but manually inspecting the toppings for each pizza can be time-consuming. Specialvideo leverages a vision AI-driven approach to detect and count ingredients in real time, making it possible for producers to uphold recipe standards, reduce waste, and maintain smooth operations.
While testing various vision solutions, Specialvideo discovered that certain techniques like semantic segmentation struggled with overlapping or hidden toppings. By using Ultralytics YOLO models, they were able to tackle these hurdles and develop a reliable, high-accuracy AI-powered quality control system that identifies, counts, and verifies toppings on the fly. It ensures consistent quality without sacrificing production speed.
Improving AI food inspection with computer vision
Founded in 1993 in Imola, Italy, Specialvideo draws on over 30 years of experience designing advanced computer vision systems for robot guidance, automated inspection, and defect detection. Their expertise also extends into computer vision in the food industry.
In particular, they developed a Vision AI food inspection system that uses instance segmentation to streamline pizza production. By treating each topping as a distinct object, the system can accurately detect, segment, and count ingredients in real time. It addresses issues like occlusions (where one ingredient is partially covered by another) and double detections (where the system identifies two instances of an ingredient when there is only one). By identifying placement errors (i.e., imbalanced toppings), manufacturers can adjust production parameters accordingly.
The model is designed to easily accommodate new ingredients and can be retrained without a full overhaul.
Also, the system can check shape, verify color compliance, and detect potential contaminants, making sure that each product meets high safety and quality standards..
Challenges in real-time food QC (quality control)
Pizza manufacturers often operate at incredibly high speeds, churning out a new pizza every 600 milliseconds. At that pace, it's very hard for human inspectors to keep up, making it challenging to monitor toppings and detect any defects accurately.
On top of that, overlapping ingredients like salami and mushrooms can obscure one another, which sometimes leads to pizzas with missing or excessive toppings, incorrect distributions, or inconsistent quantities. This not only disrupts the uniformity of the product but also harms the brand's reputation when customers receive pizzas that don’t live up to their expectations.
At the same time, these issues drive up operational costs through higher scrap rates and wasted resources. Adding to these issues, human inspectors can become fatigued over long shifts, causing their attention to wane and increasing the risk of errors.
Recognizing these pitfalls, many manufacturers are now embracing computer vision-enabled quality control and automated food inspection systems. These innovative systems provide real-time oversight and help reduce human errors while supporting high-quality outputs.
Real-time food defect detection with machine vision
Specialvideo’s Vision AI solution uses Ultralytics YOLO models' instance segmentation support to inspect every pizza in real time so that only quality products reach consumers. It accurately counts and measures toppings by comparing each pizza to its recipe, quickly detecting missing or extra ingredients, uneven distributions, and inconsistent quantities.
When a faulty pizza is detected - whether due to misaligned toppings, incorrect ingredient amounts, or contaminants like blue plastic - the system automatically diverts it to a scrap line.
To maintain accuracy post-deployment, Specialvideo continuously expands its dataset, refines labeling accuracy, and retrains YOLO regularly. They use data augmentation during training to prevent overfitting and help the model generalize from a limited number of samples. Also, 10% of the training images feature Margherita pizzas to provide helpful background context, which helps the network handle variations even within the same ingredient type, such as different kinds of salami.
Beyond this, to make the retraining process even more efficient, Specialvideo has implemented a workflow for new pizza ingredients. This workflow leverages YOLO to speed up the labeling of new images, reducing the need for human supervision as ingredient varieties evolve.
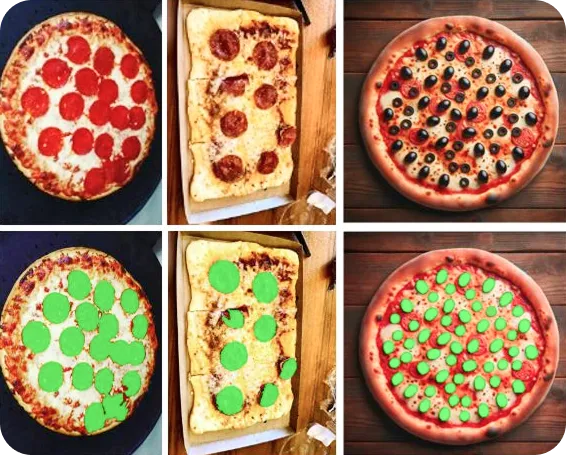
Why choose Ultralytics YOLO models?
Specialvideo chose to use Ultralytics YOLO models because they offer a great balance between AI model performance and cost. With an Nvidia GTX-1660gt GPU (Graphics Processing Unit), the system achieves inference times of just 200 - 250ms, making it fast enough to handle a production line that produces a pizza every 600ms.
YOLO’s efficient processing speed facilitates real-time quality control. Overall, this approach not only streamlines production but also supports scalability, making it a robust solution for high-volume food manufacturing environments.
YOLO-enabled food inspection solution delivers 99% accuracy
By integrating Ultralytics YOLO models, Specialvideo’s AI food inspection solution has transformed quality control in pizza production. Trained on a robust dataset of over 1,500 images, the model accurately recognizes more than 10 different ingredients, categorizing them into countable items, such as olives, salami slices, anchovies, and mozzarella balls, and non-countable items, like cubed ham, mushrooms, cheese slices, and peppers.
The YOLO-driven system operates in real-time with up to 99% accuracy, outperforming human inspectors and significantly reducing inspection times compared to manual methods.
Interestingly, the solution has demonstrated promising results by accurately recognizing ingredients in food products not included in its initial training, such as salads and pasta, facilitating easier expansion into new product lines. Ultimately, this innovative approach boosts operational efficiency, minimizes waste, and lowers costs, setting a new benchmark for automated food inspection and quality assurance.
Promoting smart manufacturing in the food industry
The road ahead for Specialvideo looks exciting. The company plans to expand its Vision AI solution to include other food products like salads and pasta. By continually fine-tuning its deep learning models and growing its dataset, Specialvideo aims to improve real-time quality control even further, reduce waste, and boost production efficiency. These enhancements will help set new industry standards.
Want to boost your operations with Vision AI? Head over to our GitHub repository to see how Ultralytics’ AI solutions are making an impact in areas like AI in healthcare and computer vision in manufacturing. Find detailed insights on our YOLO models and licensing options, and take that first step toward smarter, more efficient automation.